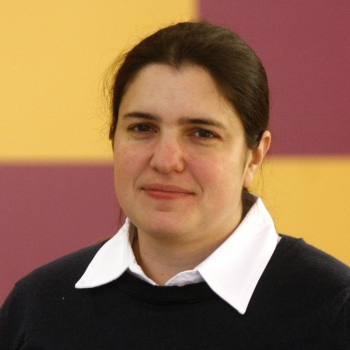
Faculty Title:
Joseph R. Mares '24 Career Development Professor in Chemical Engineering
Department:
- Chemical Engineering (ChemE)
Room:
66-464
Phone Number:
(617) 253-4584
Email:
Faculty Bio:
Professor Heather J. Kulik has been the Joseph R. Mares ’24 Career Development Chair Assistant Professor in the Department of Chemical Engineering since November 2013. She received her B.E. in Chemical Engineering from the Cooper Union for the Advancement of Science and Art in 2004 and her Ph.D. from the Department of Materials Science and Engineering at MIT in 2009. She then completed postdoctoral training in the fields of bioscience and chemistry with Felice Lightstone at Lawrence Livermore National Lab (2010) and Todd J. Martı́nez at Stanford University (2010−2013) along with a collaborative QB3 scholar appointment at UC Berkeley with Judith P. Klinman (2012-2013). Kulik’s work on electronic effects in the contribution to enzymatic rate enhancements and developments in chemical discovery and machine learning have been recognized through the Burroughs Wellcome Fund Career Award at the Scientific Interface, the 2017 I&ECR“Class of Influential Researchers”, and several invited and Editors’ Choice journal articles.
Research Areas:
Research Summary:
The Kulik Lab studies protein structure, function, and dynamics to reveal emerging contributions to enzymatic rate enhancements and understand their relationship to protein dysfunction underlying disease. This research is enabled by our developments in large-scale, fully first-principles atomistic modeling and systematic approaches for multiscale modeling to enable long-time dynamics studies of proteins. We seek to understand how the architecture of the greater protein environment controls reactivity at enzyme active sites in a fundamentally quantum mechanical fashion and to develop new computational modeling strategies that systematically and efficiently reveal residues critical to such interactions. These developments will advance computational modeling’s role in the understanding of protein function and dysfunction as well as to enable more predictive de novo protein design strategies. Current work in the group is focused on i) families of methyltransferases, which react on a wide array of different substrates and play critical roles in signaling, gene expression, and natural product synthesis as well as on ii) non-heme iron enzymes and multi-enzyme complexes involved in natural product synthesis. The group has developed new methods that only now enable us to rationalize substrate specificity as well as reveal new contributions to enzyme rate enhancement mediated by quantum mechanical charge transfer between substrates and the greater protein environment. We are now developing machine learning models that predict such key contributions only from sequence or crystal structure data, circumventing the need for direct simulation. These new techniques could also lead to more predictive paths for therapeutic inhibitor or drug design.